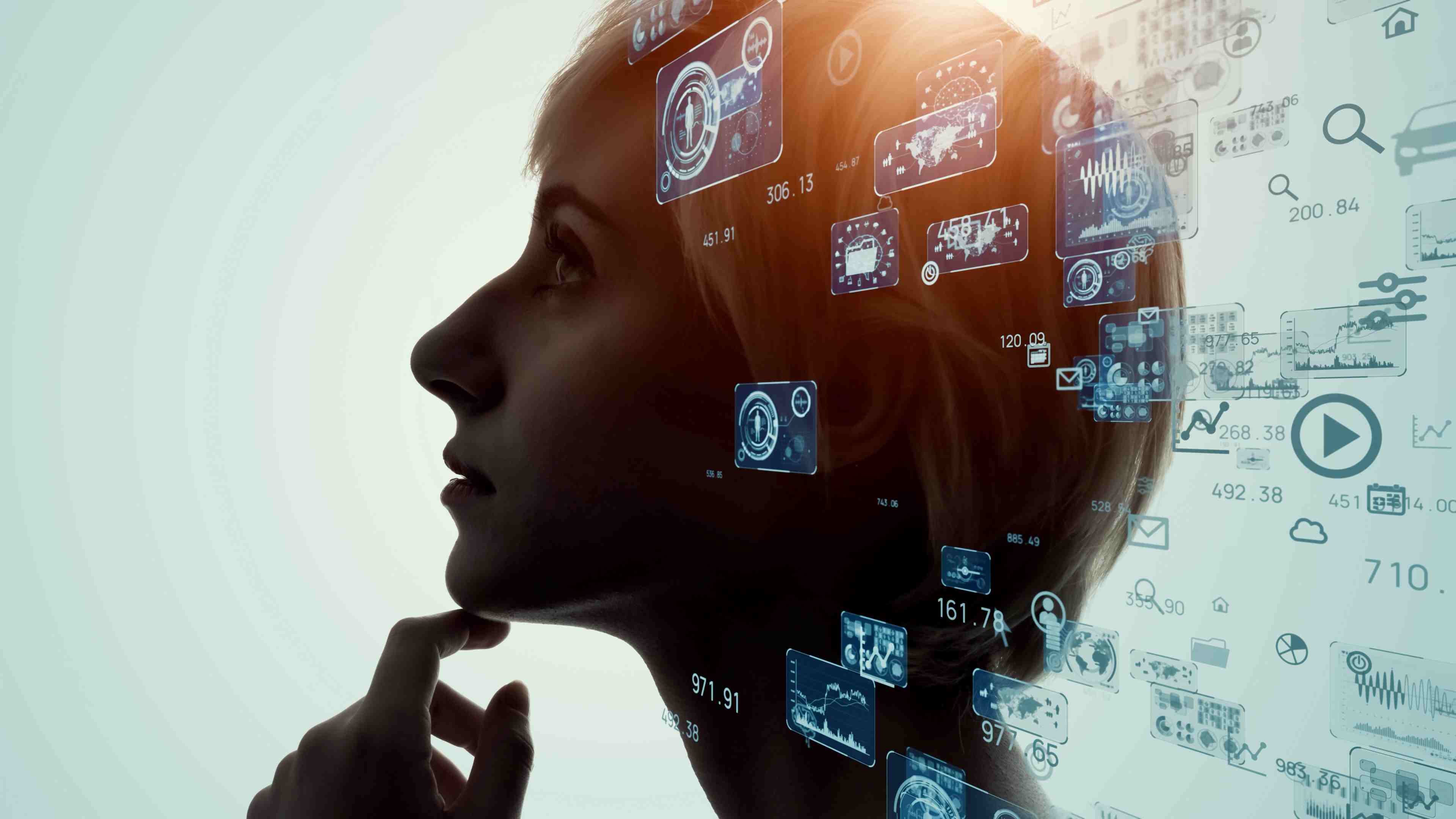
Building trust with stakeholders around AI through explainability and two-way communication
The rapid proliferation of artificial intelligence (AI) technologies has generated excitement along with apprehension. While AI promises improved efficiencies and insights, stakeholders may view unfamiliar systems as mysterious “black boxes” that fuel scepticism. To build stakeholder trust around AI initiatives, organisations need transparent communication paired with explainability.
Recent surveys reveal decreasing consumer faith in AI applications. Sixty-two percent of respondents in a 2021 study said AI made them uncomfortable because it is not transparent how the technology works. Providing clear explanations of what drives AI systems can help humanise these alien processes.
Explainable AI (or XAI) refers to methods that reveal the reasoning behind algorithmic outputs. For example, showing what data points influenced a credit approval decision or which features a facial recognition system uses to identify individuals. Explanations allow stakeholders to follow the AI’s logic.
However, explanations must avoid overly technical jargon and resonate with non-expert audiences. Using intuitive visualisations, natural language translations, or examples that connect to the stakeholder’s context improves understanding. Metaphors comparing AI processes to familiar activities makes them more accessible too.
Of course, explanations have limits. Proprietary systems maybe unable to expose protected IP, and some advanced algorithms like neural networks behave in ways even developers struggle to explain. Setting appropriate expectations is important, while finding alternative channels like showing performance metrics to build confidence.
Crucially, explanations should not overload stakeholders with too much technical detail. One study found “explanations” increased cognitive strain and decreased trust when they made concepts harder rather than easier to understand. The goal is conveying just enough to give stakeholders amental model to grasp the system’s approach on their own terms.
Explainability enables trust by signalling transparency. Stakeholders observing how AI models work internally are more inclined to trust the externally visible outputs. But the communication flow should not be one-way. organisations must also listen to stakeholder needs through two-way dialogue.
User feedback helps guide the focus of explainability efforts. What questions do people actually have about the AI? Where is clarity needed to align mental models? Structured elicitation processes like focus groups, design thinking workshops and interactive prototyping provide insights into stakeholder thinking and concerns.
Consider Microsoft’s approach when developing AI for the blind community. Through hands-on design sessions, stakeholders expressed needs to understand how computer vision algorithms categorized objects in photos. In response, researchers created explanations that addressed these user priorities rather than academic abstractions.
Ongoing communication channels allow concerns to surface early before mistrust takes root. Feedback loops improve explanations over time as organisations continuously collect stakeholder input. AI developers should view building trust as an iterative process, not a one-time exercise.
Ultimately, AI systems reflect the priorities of the people who create them. Excluding stakeholders from the development process implies their values don't matter. But by maintaining transparency through explainability and two-way communication, organisations demonstrate a commitment to addressing stakeholder concerns in good faith.
AI offers immense opportunities to transform lives, but only if governed ethically. Organisations that embrace explainability and meaningful stakeholder engagement will lead the next era of human-centred artificial intelligence.